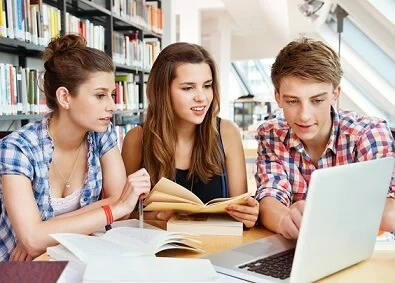
Amazon Web Services data-engineer-associate Exam Dumps
- Number 1 Amazon Web Services AWS Certified Data Engineer study material online
- Regular data-engineer-associate dumps updates for free.
- AWS Certified Data Engineer - Associate (DEA-C01) Practice exam questions with their answers and explaination.
- Our commitment to your success continues through your exam with 24/7 support.
- Free data-engineer-associate exam dumps updates for 90 days
- 97% more cost effective than traditional training
- AWS Certified Data Engineer - Associate (DEA-C01) Practice test to boost your knowledge
- 100% correct AWS Certified Data Engineer questions answers compiled by senior IT professionals
Amazon Web Services data-engineer-associate Braindumps
Realbraindumps.com is providing AWS Certified Data Engineer data-engineer-associate braindumps which are accurate and of high-quality verified by the team of experts. The Amazon Web Services data-engineer-associate dumps are comprised of AWS Certified Data Engineer - Associate (DEA-C01) questions answers available in printable PDF files and online practice test formats. Our best recommended and an economical package is AWS Certified Data Engineer PDF file + test engine discount package along with 3 months free updates of data-engineer-associate exam questions. We have compiled AWS Certified Data Engineer exam dumps question answers pdf file for you so that you can easily prepare for your exam. Our Amazon Web Services braindumps will help you in exam. Obtaining valuable professional Amazon Web Services AWS Certified Data Engineer certifications with data-engineer-associate exam questions answers will always be beneficial to IT professionals by enhancing their knowledge and boosting their career.
Yes, really its not as tougher as before. Websites like Realbraindumps.com are playing a significant role to make this possible in this competitive world to pass exams with help of AWS Certified Data Engineer data-engineer-associate dumps questions. We are here to encourage your ambition and helping you in all possible ways. Our excellent and incomparable Amazon Web Services AWS Certified Data Engineer - Associate (DEA-C01) exam questions answers study material will help you to get through your certification data-engineer-associate exam braindumps in the first attempt.
Pass Exam With Amazon Web Services AWS Certified Data Engineer Dumps. We at Realbraindumps are committed to provide you AWS Certified Data Engineer - Associate (DEA-C01) braindumps questions answers online. We recommend you to prepare from our study material and boost your knowledge. You can also get discount on our Amazon Web Services data-engineer-associate dumps. Just talk with our support representatives and ask for special discount on AWS Certified Data Engineer exam braindumps. We have latest data-engineer-associate exam dumps having all Amazon Web Services AWS Certified Data Engineer - Associate (DEA-C01) dumps questions written to the highest standards of technical accuracy and can be instantly downloaded and accessed by the candidates when once purchased. Practicing Online AWS Certified Data Engineer data-engineer-associate braindumps will help you to get wholly prepared and familiar with the real exam condition. Free AWS Certified Data Engineer exam braindumps demos are available for your satisfaction before purchase order.
- Target Audience: This exam is geared towards data engineers with 2-3 years of experience and 1-2 years of hands-on experience with AWS.
- Exam Format: You will face 65 questions (50 scored and 15 unscored) in a pass/fail format. AWS uses unscored questions to gauge the effectiveness of future exams.
Exam Content: The exam covers a broad range of topics, including:
- We design and implement data pipelines using AWS services like Glue, Lambda, and Step Functions.
- Choosing the right data store (S3, DynamoDB, Redshift, etc.) based on data characteristics and access patterns.
- We are designing data models and ensuring data quality throughout the pipeline.
- We are monitoring and troubleshooting data pipelines for optimal performance and cost efficiency.
To ace the exam, a comprehensive study plan is crucial. Here are some valuable resources:
Send us mail if you want to check Amazon Web Services data-engineer-associate AWS Certified Data Engineer - Associate (DEA-C01) DEMO before your purchase and our support team will send you in email.
If you don't find your dumps here then you can request what you need and we shall provide it to you.
Bulk Packages
3 Exams PDF
- Get 3 Exams PDF
- Get $33 Discount
- Mention Exam Codes in Payment Description.
5 Exams PDF
- Get 5 Exams PDF
- Get $65 Discount
- Mention Exam Codes in Payment Description.
5 PDF + Engine
- Get 5 Exams PDF + Test Engine
- Get $105 Discount
- Mention Exam Codes in Payment Description.
10 PDF + Engine
- Get 10 Exams PDF + Test Engine
- Get $280 Discount
- Mention Exam Codes in Payment Description.
AWS Certified Data Engineer
We are providing Amazon Web Services data-engineer-associate Braindumps with practice exam question answers. These will help you to prepare your AWS Certified Data Engineer - Associate (DEA-C01) exam. Buy AWS Certified Data Engineer data-engineer-associate dumps and boost your knowledge.
The exam is designed to validate skills in designing, building, securing, and maintaining analytics solutions on AWS for individuals with experience in data engineering roles.
The exam covers various domains related to data engineering on AWS, including data collection, storage, processing, and visualization, utilizing services like Amazon S3, Amazon Redshift, Amazon DynamoDB, Amazon EMR, AWS Glue, Amazon Kinesis, and more.
While there are no mandatory prerequisites, candidates must have at least two years of experience with AWS technology, proficiency in programming languages, and familiarity with AWS security best practices.
The exam consists of multiple-choice and multiple-answer questions, assessing candidates' ability to apply AWS data services to derive insights from data.
Candidates can prepare using resources provided by AWS, such as training courses, whitepapers, FAQs, and documentation. Practice exams and study guides are also available to help understand the exam format.
The certification is valid for three years from the date of issuance.
To maintain certification status, professionals must recertify by either passing a recertification exam or advancing to a higher level of certification.
Data engineers seeking to prove their skills in cloud data engineering and advance their career opportunities can benefit from obtaining this certification.
Services such as Amazon S3, Amazon Redshift, Amazon DynamoDB, Amazon EMR, AWS Glue, and Amazon Kinesis are covered in the exam.
The certification demonstrates expertise in designing, building,
securing, and maintaining analytics solutions on AWS that are efficient,
cost-effective, and scalable.